The Math Behind Stopping Ebola
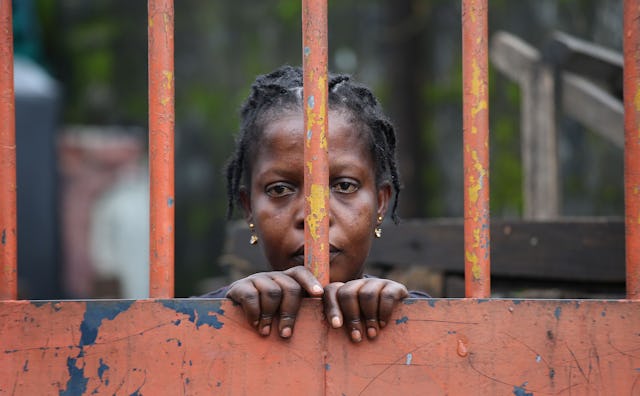
The word is almost onomatopoeic. Ebola. Something thick and undulant; like those snakelike electron micrographs of the virus, like the Ebola River after which it was named, like the blue-black bruises that can mark the late, hemorrhagic stages of the disease. In 1976, the first outbreak of Ebola killed 88 percent of the people it infected. Bubonic plague’s fatality rate is lower by one-third. When researchers named the Ebola virus, they chose carefully, picking the nearby river as eponym instead of the closest town, for fear of bringing infamy to the village. In Lingala, the word means “black.” In English, it means fear.
The management of this fear—and indeed, of the disease itself—is a delicate, bitterly complex endeavor. President Obama’s appointment of Ron Klain as ‘Ebola czar’ is proof enough of the bureaucratic hurdles inherent to the United States’ domestic and international responses to the disease. Klain is a previous Chief of Staff to Vice Presidents Al Gore and Joe Biden. He knows how to handle bureaucratic hurdles. What he doesn’t know is how to stop Ebola.
That job falls to an elaborate web of government officials, healthcare leaders, and academic researchers woven through the public, non-governmental, and academic spheres. It may be Klain’s position to serve as a coordinating spider of sorts on this web, but it is the other players, working at organizations like the Centers for Disease Control and Prevention and the World Health Organization, who are in the real business of halting the spread of the disease. At the root of their work is a set of three basic questions about the global situation: How bad is it, how bad will it get, and what should we do to stop it?
It’s bad. The current outbreak of Ebola virus disease has killed more people than all previous outbreaks combined. At the time of writing, there have been almost 10,000 cases of the disease in West Africa, with the number doubling about every three weeks.
©Bex Levine (CDC Global/flickr)
As for the other two questions, the only way to get any semblance of a handle on the future of the current outbreak is to turn a meticulous eye to the past. Doing so ushers us into the world of mathematical epidemiology, in which computational modelers strive to guide public health efforts by studying past outbreaks of a disease. It’s a tricky job, and one that requires a number of simplifying assumptions. With respect to the current Ebola epidemic, part of the difficulty is that there has never been an outbreak like this before. Past Ebola outbreaks have been relatively small and limited to rural areas with low population densities. It’s when the virus wanders into Monrovia—the Liberian capital with fifteen ambulances and four treatment clinics serving a population of one million—that extrapolation from a model based on 318 cases becomes difficult. But it’s what we have.
Back To the Future
Broadly speaking, studying past outbreaks of Ebola is immediately useful for two reasons: It helps us estimate the amount of resources we’ll need to combat the current outbreak, and it suggests where we should direct them. That is, it answers the questions of how bad it will get and what we should do. One goal of model design is to estimate the impact of possible public health interventions on control of the disease. By getting a quantitative grip on the effectiveness of past control measures, we have a better chance of choosing the most appropriate future interventions.
Many disciplines have a canonical number: something that anchors discussion and provides a reference point for many comparisons. For economics, the number is GDP. For infectious disease epidemiology, it is R0, the basic reproductive number (pronounced “R-nought”). The number provides a measure of the communicability of a given disease—the average number of secondary cases that result from one infection. An R0 of one denotes a steady state in which a disease neither grows nor diminishes. Values below one indicate the petering out of disease; values above one imply an epidemic. Highly infectious diseases like measles and pertussis have R0s in the double digits. For the current Ebola outbreak, the value seems to be somewhere between 1.5 and 2.5.
That people die relatively quickly is a beneficial tragedy in terms of Ebola’s spread.
Which doesn’t sound so bad. But keep in mind that an R0 above one implies exponential growth. When paired with a high mortality rate, the effect can be devastating. Chickenpox spreads around kindergarten pretty quickly, but kids don’t die from it. When it is fatal, the Ebola time course is sharp and severe: nine or ten days of incubation, a week of symptom presentation, and death. That people die relatively quickly is a beneficial tragedy in terms of Ebola’s spread. A longer time course would almost certainly give rise to a higher R0.
By modeling communicability over time, researchers can measure the effects of various control measures. Estimating a reproductive number at each point in time (at each day of an epidemic, for example) gives rise to a shifting stream of communicability rates called Rt. If a modeler wants to measure the effect of, say, delivering an education campaign, she can overlay the dates of the intervention onto the changing Rt values. A reduction in Rt doesn’t necessarily mean the intervention was successful—this is the old fallacy of correlation versus causation—but modelers have an arsenal of mathematical controls for getting closer to the truth.
Quarantines, Contact Tracing, and Travel Bans
Moving from models to actionable steps, however, takes us through a thorny mathematical forest. At its core, a given model derives R0 and a stream of Rt’s from a series of features that describe the course of the disease in a population. If a modeler can calculate a daily transmission rate in a variety of settings (e.g. in the community, in a hospital, etc.) and the infectious duration of a disease, she can calculate R0. In practice, it’s hideously difficult to do this accurately, in part because researchers are often only left with a list of times of diagnoses and deaths. The most common epidemiological model of choice is an SEIR model, in which each letter of the acronym denotes a subgroup of the population: susceptible, exposed, infectious, and recovered. In an SEIR model, members of a population pass from group to group at rates based on the available data.
One of the great things about these models is that they’re probabilistic. A modeler can specify, for example, the rate at which a doctor accidentally—randomly—pricks himself with an infectious needle. (That’s one more unit shifted from the susceptible to the exposed group.) More parameters means bigger computational slogs but higher predictive power. Indeed, the most complete models are those that reflect the real world in all its uncertain glory. Misdiagnosis, delays in detection, and a lack of epidemiological surveillance systems are all parts of this world. Healthcare is imperfect; it’s the modeler’s job to take these reality checks into account.
It’s also in this real world of imperfect healthcare that policymakers need to make real decisions about quarantining, contact tracing, travel bans, and other ethically squishy control phenomena. It’s self-evident that perfect quarantining and contact tracing would stop a disease in its tracks. But “perfect” suggests an idealism that overshoots the reality of many West African healthcare infrastructures. It’s also not strictly necessary according to the math. To contain Ebola, we need to push the R0 from around two to below one. What this actually translates to, all else held constant, is an intervention or series of interventions that’s 50 percent effective. A vaccine that only protects half the population could curtail the spread of the disease.
A model of the current outbreak by Cameron Browne of the University of Vanderbilt and colleagues stresses that if we want to have any shot at containing Ebola in West Africa, we need to get the time from symptom appearance to diagnosis down to about three days. Furthermore, the authors suggest that to achieve containment within a relatively short time span, the probability that someone who has had contact with an infected person is isolated without causing another case needs to be around 50 percent.
This means education campaigns, better epidemiological surveillance, and more community health workers; a call echoed by an October 2014 review of Ebola transmission dynamics by Gerardo Chowell of Arizona State University and Hiroshi Nishiura of the University of Tokyo. It means diagnostic kits that can identify Ebola before the symptoms set in.
Airport screenings are ineffective for a number of reasons, perhaps most glaringly exemplified in the case of a Canadian report on airport screening during the 2003 SARS epidemic, which concluded that despite 6.5 million Canadian “screening transactions,” no cases were detected. SARS, like Ebola, has a moderately long incubation period, and analysis of probable SARS cases showed that travelers to Canada “became ill after arrival and would not have been detected by airport screening measures.”
Travel bans, too, can be particularly dangerous to public health and epidemiological efforts, since some of our most valuable data for mapping the potential spread of Ebola are based on current, uninterrupted travel trends. Cutting off specific air travel routes won’t necessarily stop people from moving, but it will make their movement harder to track and nearly impossible to predict. Besides, under a travel ban, medical aid workers wouldn’t be able to fly to where they’re needed most. In practice, travel bans can generate panic and ostracize an entire continent. Which brings us back to the fear.
Panic At Home
October 15, 2014, a Wednesday. In the video, the second healthcare worker from Texas Health Presbyterian is arriving in Atlanta. Count the vehicles: one private jet, one ambulance, a motorcade with ten sets of flashing lights. Footage of the nurse in a yellow hazmat suit, what look like bags on her feet; uncertain steps, she cannot see. Escorted by two hazmats that match one another but not her. Recounting her temperature, her phone call, her flight; not her name. Footage of the motorcade. Anderson Cooper: She called the CDC to fly and got the okay to go. Cut to CDC Director: “She should not have traveled on a commercial airline.”
The cracks are appearing.
In the U.S., we’re somewhere on a shaky spectrum between anxious and nuclear panic. Some of it is pre-election politicization; some of it is utter zaniness. Sanjay Gupta is demonstrating how to properly remove personal protective gear, a woman flying out of Dulles is doing so in a homemade hazmat suit, at least six schools are closing in Texas and Ohio, and somehow, in one of the finer displays of journalistic integrity this side of Ed Murrow, it is Shep Smith at Fox News stepping in with a sobering plea to stop the madness.
Under the World Bank’s worst-case scenario, Liberia could lose up to 12 percent of its 2015 GDP.
This is a game of language and imagery, and the rhetoric of the Ebola response is one of euphemism and deflection. We talk of porous borders, controlled movement, draining the reservoir, the hot bed, dead-body-management teams. The language is useful as a diversion (and for ratings), but less so for coming to terms with the facts. It also rejects the notion that Ebola is a disease that affects real people and families.
Functioning at the population level, the world of mathematical epidemiology is similarly indifferent to individual life, but the indifference seems more useful here. When the game is statistical, it’s possible to find consolation in this uncertainty, not cower in its wake. The function of mathematical models, then, is twofold: Not only do they help us piece together a response, but they help us talk about it rationally. There’s comfort in the math: a sense that we’ve been here before. That this will get worse before it gets better, but yes, it will get better. In the meantime, how many rubber gloves do we need in Guinea? Panic is almost never rational. Math almost always is.
©Athalia Christie (CDC Global/flickr)
Picking Up the Pieces
“We have to rethink the way we address Ebola infection control,” said Tom Frieden, the Director of the CDC, at a recent press conference, “because even a single infection is unacceptable.”
We are rethinking Ebola control. Nearly every week, epidemiologists develop new models, the CDC releases new guidance, and the WHO releases new targets. Understanding disease, like many other things, is about understanding its patterns. The scale of these patterns varies astronomically. Mathematical epidemiology attempts to model gross changes in transmission rates and susceptibility—but it doesn’t operate in a vacuum. On the other end of the spectrum is the field of genomics, tasked with analyzing individual differences in viral genomes across patients and measuring the extent to which the virus evolves as it spreads.
This is still to say nothing of the economic models at play. The World Bank put a $32.6 billion price tag on their worst-case scenario, a situation in which containment mistakes lead to 200,000 total cases of Ebola. (Compare these figures to the CDC’s worst-case scenario of a total of 1.4 million cases in Liberia and Sierra Leone by the end of January 2015. The potential cost becomes staggering.) For the West African nations hit the hardest, the economic effects are dire: Under the World Bank’s same worst-case scenario, for example, Liberia could lose up to 12 percent of its 2015 GDP. It’s these kind of cost-benefit analyses that make early investment in Ebola containment a no-brainer.
Linking up the various models is crucial for containing the disease because it helps us paint a more convincing picture than any single model could paint alone—one that is realistic, actionable, and pressing. We can’t expect an epidemiological model to tell us anything accurate if we’re actually talking about two genetically distinct diseases, and we can’t expect our biological models to translate into action if they don’t have economic models through which to filter. As of October 22, Liberia had 620 beds in Ebola treatment units but needed 2,690. A 10,000-bed treatment facility would require as much as $200 million per month to function. By the same logic, a 100,000-bed effort would need $2 billion.
For epidemiological modelers, the difference between 10,000 and 100,000 cases is a line of code. This fact is useful for decision-making in a crisis, but there is indeed a danger lying in wait: the threat of desensitization, of becoming too far removed. “Don’t panic” cannot mean “don’t empathize.” Calming down cannot mean subduing urgency. Cross-disciplinary collaboration, then, can act as a mechanism to keep that urgency in just the right place: stretched and spread across the web of global actors, but intact.
Cover Photo: John Moore/Getty Images
This article was originally published on